Research from NIEHS intramural scientists and colleagues shows that artificial intelligence (AI) and machine learning can effectively detect and diagnose polycystic ovary syndrome (PCOS), the most common hormone disorder among women aged 15 to 45. The researchers systematically reviewed published scientific studies that used AI and machine learning to analyze data to diagnose and classify PCOS. They found that AI and machine learning-based programs were successful in detecting PCOS.
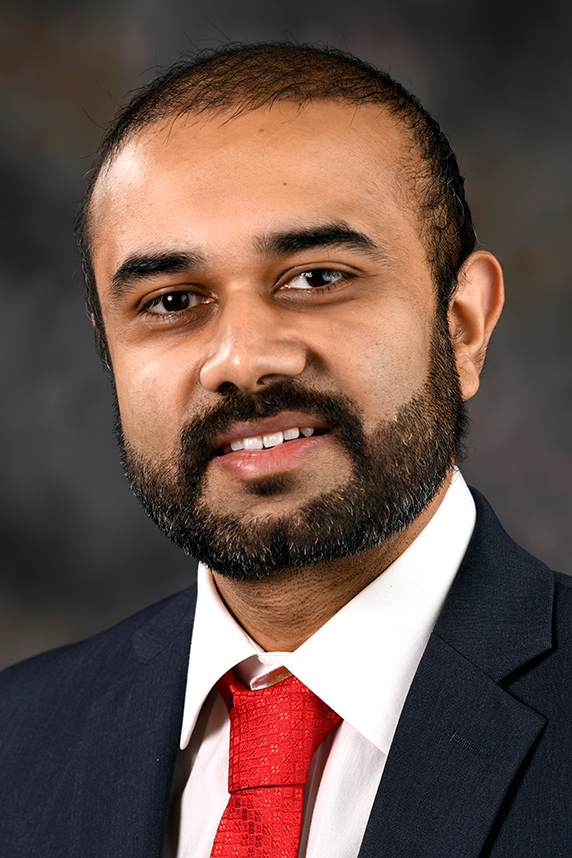
“Given the large burden of under- and misdiagnosed PCOS in the community and its potentially serious outcomes, we wanted to identify the utility of AI and machine learning in the identification of patients who may be at risk for PCOS,” said Janet Hall, M.D., NIEHS senior investigator and endocrinologist, and a study co-author. “The effectiveness of AI and machine learning in detecting PCOS was even more impressive than we had thought.”
Connecting the dots
PCOS occurs when the ovaries do not work properly and, in many cases, is accompanied by elevated levels of testosterone. The disorder can cause irregular periods, acne, extra facial hair, or hair loss from the head. Women with PCOS are often at an increased risk for developing type 2 diabetes, as well as sleep, psychological, cardiovascular, and other reproductive disorders such as uterine cancer and infertility.
“PCOS can be challenging to diagnose given its overlap with other conditions,” said Skand Shekhar, M.D., senior author of the study and assistant research physician and endocrinologist at the NIEHS. “These data reflect the untapped potential of incorporating AI and machine learning in electronic health records and other clinical settings to improve the diagnosis and care of women with PCOS.”
PCOS diagnosis is based on widely accepted standardized criteria that have evolved over the years, but typically includes clinical features (e.g., acne, excess hair growth, and irregular periods) accompanied by laboratory (e.g., high blood testosterone) and radiological findings (e.g., multiple small cysts and increased ovarian volume on ovarian ultrasound). However, because some of the features of PCOS can co-occur with other disorders, such as obesity, diabetes, and cardiometabolic disorders, it frequently goes unrecognized.
A systematic review of PCOS diagnoses
AI refers to the use of computer-based systems or tools to simulate human intelligence and help make decisions or predictions. Machine learning is a subdivision of AI focused on learning from previous events and applying this knowledge to future decision-making. AI can process massive amounts of distinct data, such as that derived from electronic health records, making it an ideal aid in difficult to diagnose disorders like PCOS.
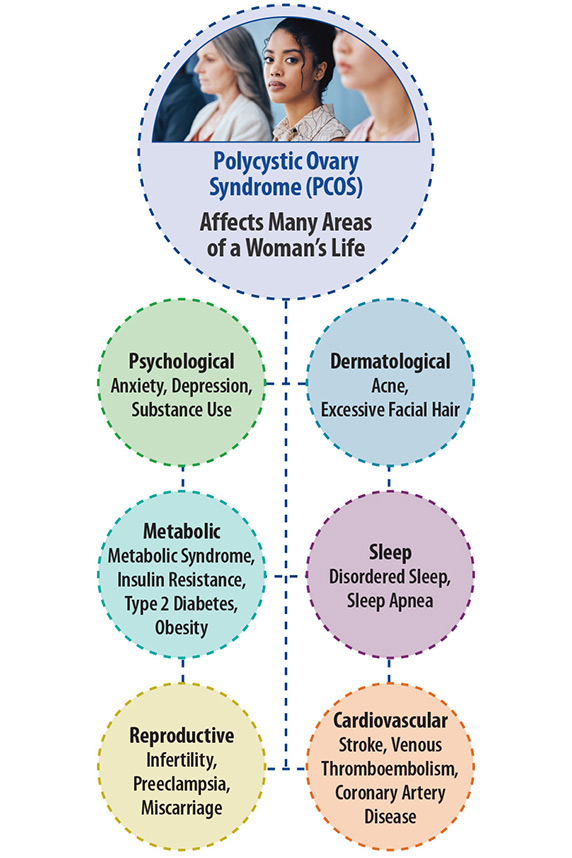
The researchers conducted a systematic review of all peer-reviewed studies published on this topic for the past 25 years (1997-2022) that used AI and machine learning to detect PCOS. They screened 135 studies and included 31 in this paper. All studies were observational and assessed the use of AI and machine learning technologies on patient diagnosis. Among the 10 studies that used standardized diagnostic criteria to diagnose PCOS, the accuracy of detection ranged from 80-90%.
“Across a range of diagnostic and classification modalities, there was an extremely high performance of AI and machine learning in detecting PCOS, which is the most important takeaway of our study,” said Shekhar.
The authors note that AI and machine learning-based programs have the potential to significantly enhance early detection of PCOS in women, with associated cost savings and a reduced burden on patients and the health care system.
Follow-up studies with robust validation and testing practices will allow for the smooth integration of AI and machine learning for chronic health conditions.
Congressional briefing
After the press release about the paper came out, it caught the attention of stakeholders headed to Capitol Hill. Shekhar was invited to share the new findings at a congressional briefing on PCOS. The briefing was hosted by the Bipartisan Congressional PCOS Caucus, Congresswoman Debbie Wasserman Schultz (D-FL-25), and Congressman David Scott (D-GA-13). The event was sponsored by the PCOS Challenge: The National Polycystic Ovary Syndrome Association on Sept. 26 at the Cannon House Office Building in Washington, D.C.
(Robin Mackar is a writer and media relations coordinator in the NIEHS Office of Communications and Public Liaison.)